Media | Articles
Stanford and Toyota are sending AI to drifting school—to save your life
As we move towards a reality in which autonomous vehicles share the roads with human-driven ones, automakers are looking at every potential sort of learning material to train and program their artificially intelligent systems. They’re even taking some lessons from those sideways hooligans in drifting.
“Since 2008, our lab has taken inspiration from human race car drivers in designing algorithms that enable automated vehicles to handle the most challenging emergencies,” says Professor Chris Gerdes of Stanford University’s Dynamic Design Laboratory. The department is best known for Marty, the self-drifting DeLorean DMC-12.
“Through this research, we have the opportunity to move these ideas much closer to saving lives on the road,” he continues. To realize Toyota’s lofty goal of zero traffic fatalities, vehicle controls must become increasingly active in the decision-making process that determines throttle, braking, and steering inputs during an emergency maneuver.
Traditional traction control has carried a buzz-kill reputation because it does little more than bring you back in line. Such systems focus on cutting power to stop wheel spin, braking individual tires in order to jerk the car back on course, and, with the rise of electronic power steering, even steering the wheel to nudge the car into the center of a lane. More often than not, however, these system are simply reactive measures with a one-track mind: Stop the slide.
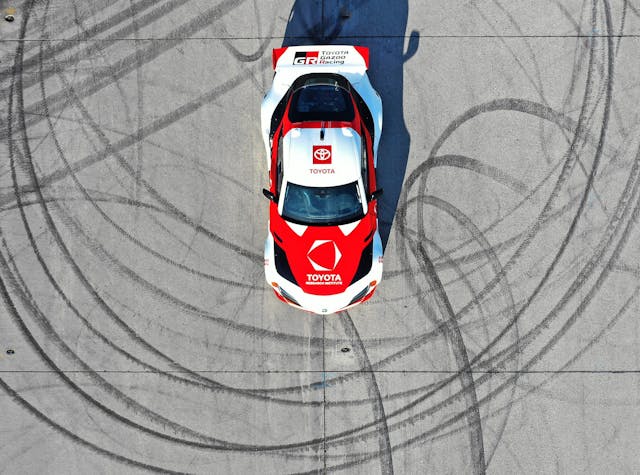
Stanford’s Dynamic Design Laboratory and TRI change the script by introducing more advanced car control techniques to their stability and autonomous driving systems, teaching the car that a bit of slip-angle may be the better move in certain situations. The average driver easily outreaches their skill sets—or simply human perception—when desperately attempting to evade a crash, and AI might be able to lend a professionally “trained” hand.
Marketplace
Buy and sell classics with confidence
“Every day, there are deadly vehicle crashes that result from extreme situations where most drivers would need superhuman skills to avoid a collision,” says Gill Pratt, CEO of Toyota Research Institute (TRI) and chief scientist at Toyota Motor Corporation. “The reality is that every driver has vulnerabilities, and to avoid a crash, drivers often need to make maneuvers that are beyond their abilities. Through this project, TRI will learn from some of the most skilled drivers in the world to develop sophisticated control algorithms that amplify human driving abilities and keep people safe. This is the essence of the Toyota Guardian™ approach.”
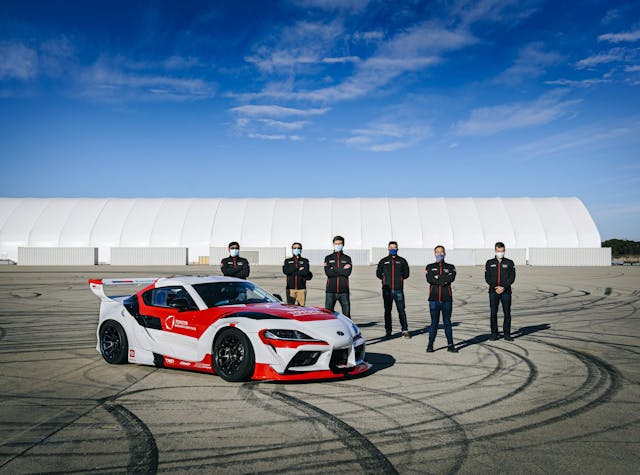
Extreme car control has been absent from most autonomous driving routines because the necessary decision-making process is a computationally expensive one. In a fraction of a second, the vehicle must perceive a list of variables, spit out the right commands to initiate a drift (if that were determined to be the best move), and potentially maintain the slide while the vehicle’s chassis regains its equilibrium.
We humans, through experience and training, develop a muscle memory that helps determine the ideal trajectory before we recover a slide. Could a traction-control system could replicate that learning process? Well, that’s where Stanford’s research comes into play.
The Dynamic Design Laboratory focuses on developing a computer algorithm that creates a feedback loop to measure vehicle stability and the driver’s intentions, working between them to make input changes while prioritizing the slip-angle of the tires. This feedback loop behaves not unlike OBDII, which has open- and closed-loop routines that vary based on vehicle conditions.
“Open loop” means that the vehicle’s computer is reading sensors and deciding its next move based on the sensor array; “closed-loop” refers to a system that has essentially entered a prescheduled routine with stricter operating parameters. The first is similar to the mental process that occurs when you encounter a wet patch of road, detect that grip has diminished, and then adjust your inputs. The second is similar to prior knowledge: You know ahead of time that the road is and preemptively drive carefully.
Stanford’s algorithm aims to expand this computational process. First, the algorithm takes a snapshot of the vehicle’s situation, simulates a multitude of reactions, and chooses the best result. Then, the algorithm figures out how to manipulate the vehicle to perform the chosen behavior.
For Stanford and Toyota, the drifting demonstrations are for more than just great PR videos. Controlling a prolonged slide remains one of the most challenging tasks for a driver of any kind, whether artificially or organically intelligent, and a successfully balancing the car on the limit of grip is a triumph of (digital) mind over matter.